Ultralow-dose CT Scan Promises Better Resolution, Less Radiation than Conventional Method
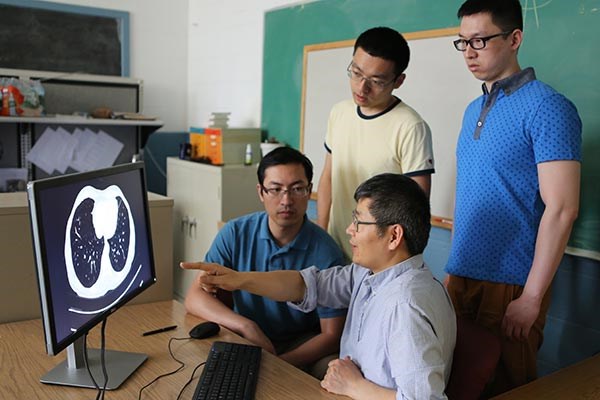
11/01/2016
By Edwin L. Aguirre
Lung cancer is the leading cause of cancer death in the United States, with a five-year relative survival rate of only 15.8 percent for smokers and non-smokers combined, according to the U.S. Centers for Disease Control and Prevention. Most lung cancer does not cause any symptoms until the disease has spread. That is why detecting it early, when treatment is more likely to be effective, is of utmost importance.
Diagnostic tools that physicians use to conduct initial tests for lung cancer can include chest X-rays and low-dose computed tomography (CT) scans. Unlike a regular chest X-ray, which takes a single two-dimensional image at a time, the CT scanner takes multiple X-ray images as it rotates around the patient lying on a table. A computer then combines the images to create a detailed, three-dimensional cross-section of the patient’s body. The downside is that the procedure exposes the patient to a higher dose of radiation than the chest X-ray.
A team of researchers led by electrical and computer engineering Assoc. Prof. Hengyong Yu is developing a new ultralow-dose CT screening method to improve the image quality and resolution of CT scans while reducing the radiation dose.
Higher quality and resolution means the CT images would enable doctors to determine more precisely the size, shape and position of lung tumors and help locate enlarged lymph nodes that might contain cancerous cells that have spread from the lungs. Lower X-ray dose means the patient will be exposed less to ionizing radiation, which can damage healthy cells and increase the risk for malignancy.
“Currently, the average effective radiation dose of a low-dose CT scan for lung cancer screening for an adult is about 1.5 millisieverts. Our goal is to reduce the dose to less than 0.5 millisievert, which is comparable to a single radiographic chest X-ray,” explains Yu.
To accomplish this, Yu and his group are combining state-of-the-art biomedical imaging techniques called “statistical iterative reconstruction” and “dictionary learning.” Statistical iterative reconstruction uses special algorithms and statistical modeling to reconstruct 2-D and 3-D scans of the lungs with less image artifacts, even when the raw data is noisy or incomplete. Dictionary learning uses mathematical signal processing to remove noise and improve image quality.
“Our method will benefit the more than 90 million current and former smokers in the country who are at high risk for lung cancer,” notes Yu.
Reducing Treatment Cost and Anxiety
Yu points out that over-diagnosis incurs unnecessary follow-up treatment, cost and anxiety to patients.
In the National Lung Screening Trial (NLST), low-dose CT images that reveal any non-calcified nodule of greater than 4 millimeters in size were classified as positive, or suspicious for lung cancer. “Other abnormalities such as adenopathy or effusion were classified as positive as well,” he says.
With low-dose CT, the rate of positive screening tests in the trial was 24.2 percent, and 96.4 percent of those positive tests were “false positives.” Nodules between 4 mm and 1 centimeter in size were followed up with repeated CT scanning over the next three to six months. Biopsies were also recommended for nodules larger than 1 cm.
In response to this over-diagnosis, Yu is working on another research project to develop novel imaging biomarkers so that the rate of false positives could be greatly reduced while maintaining the rate of “false negatives” to current levels.
“The key is to explore hidden information in the CT images from the NLST database using cutting-edge deep machine learning techniques and algorithms,” he says.
Yu’s research is funded by the National Science Foundation and the National Institutes of Health, with grants totaling more than $1 million. His collaborators include computer science Assoc. Prof. Yu Cao and electrical and computer engineering Prof. Yan Luo, as well as clinical physicians from Massachusetts General Hospital, medical physicists from Stanford University, biomedical imaging experts from Rensselaer Polytechnic Institute, algorithmic mathematicians from the University of Central Florida and industry leaders from GE Global Research Center.