Assoc. Prof. Ann Kronrod Develops Algorithm to Analyze Language of Online Reviews
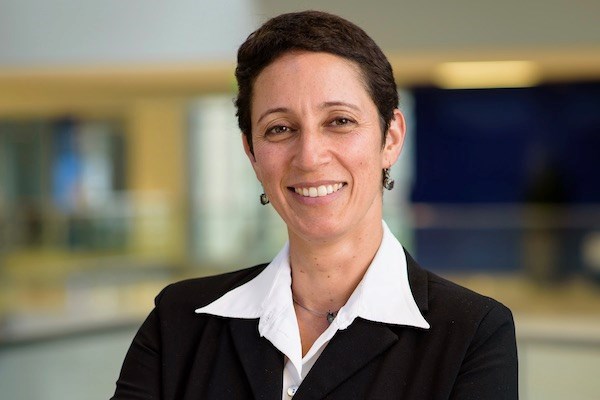
Assoc. Prof. of Marketing Ann Kronrod's paper on how episodic and semantic memory affects the language of authentic and fictitious reviews online was recently published in the Journal of Consumer Research.
12/22/2022
By Ed Brennen
But how do you know if the review you are reading is real and not a work of fiction?
Ann Kronrod, an associate professor of marketing, entrepreneurship and innovation (MEI) in the Manning School of Business, is developing a tool to help you find out.
Kronrod, whose research interests include linguistics and text analysis in the marketing arena, has examined how the language used in an online review can reveal whether or not the reviewer actually tried the product — and has created an algorithm that can help flag fake reviews.
She is the lead author of a recently published paper in the Journal of Consumer Research titled “Been There, Done That: How Episodic and Semantic Memory Affects the Language of Authentic and Fictitious Reviews.”
“People want to know if what they are reading is genuine,” says Kronrod, who is conducting the research with two assistant professors of marketing, Jeffrey K. Lee of American University in Washington, D.C., and Ivan Gordeliy of the EDHEC Business School in France.
Businesses also have a vested interest in legitimate reviews, Kronrod says.
“If consumers buy a product and it’s different from what was in the review, they’re angry with the company. So it’s the company’s problem,” she says.
Born in Russia and raised in Israel, Kronrod has had a lifelong love for language (she’s fluent in English, Russian and Hebrew and can also speak French, Italian, Arabic and Spanish). Applying linguistics to the business world, she says, “is a perfect combination of creativity, strategy and philosophy.”
Kronrod sat down recently to discuss her research.
Q: How big of a problem are fake reviews?
A: There are alarming statistics about what percentage of the reviews that we read are not genuinely by consumers who tried the product. For example, Amazon reports flagging about 70% of the reviews posted on its platform. Even the reviews marked as a “verified purchase” can be faked.
Q: Why would someone write a fake review?
A: Most of these reviews are being ordered, or invited, by companies. The company gives you the product to try for free and asks you to write a review. In many cases, though, the request is not, “Leave us a review,” it is, “Leave us a positive review.” And this immediately sways and biases the consumer. But that's not what my colleagues and I are looking for. We're looking for indicators of whether people tried the product or not. Leaving a review for a product you haven't tried is, by definition, a lie.
Q: Your paper talks about episodic versus semantic memory. What does that mean?
A: If you tried a product, then you will have specific memories of things that happened while you tried it. So, for example, when you talk about your last vacation, you will be telling about people you met or things that you saw, and these would be things that you gather from your episodic memory because it's a memory of episodes during your experience.
But if you're trying to talk about your last flight to the moon, that would be something you haven't probably tried. You don't have an episodic memory of that experience. What you do have is memory of facts from other people’s descriptions of such experiences, called semantic memory, and that's where you're going to derive your language from. You're going to describe the views, the zero gravity, the things that you remember from other people’s accounts or maybe of what you’ve seen in the movies.
Our suggestion is that, other things being equal, these two types of memory will make your language fundamentally different in various ways.
Q: What are those ways?
A: Concreteness is one; people will be more concrete if they have real memories of an experience. For example, they’ll say “apple” instead of “fruit.” They will also use more words that are unique to the domain and less general words.
We also noticed that in fake reviews, people reuse the same general words over and over again. So, if you're describing a hotel in which you didn't stay, you would repeat the word “hotel” because you don't have other words in your memory. There’s less variety of language in fake reviews.
Fake reviews use less content words, which are nouns, verbs, adjectives and adverbs. Instead, they either recycle the same word or use lots of connection words like “very, very.” They also repeat words from the instructions they are given for the review.
Q: What type of experiments have you conducted?
A: We ran an experiment with 800 people where half the people tried a product that we created — a phone app with neck stretching exercises. The other half did not try the app; we just told them that there is this product that we created. Then we asked everyone to write reviews of the app, which we harvested and used to analyze the linguistic features. So, half of the people wrote reviews about the app after trying it, and the other half wrote reviews without trying the app.
From there, we wrote a code and developed an algorithm that taught the computer how to distinguish between real and fake reviews using these linguistic features. We then tested our algorithm on two datasets of published reviews, for example a set of reviews that were downloaded from Amazon.
Q: What’s next for this research?
A: Several things. First, a large retailer contacted us and asked if we would work with them and tell them which reviews are real and fake on their website. They have millions of reviews, and hopefully we can implement our algorithm and help the company.
Another direction that this collaboration might take is testing the reviews that are left by the sellers themselves on the company’s website. It's not like they don't know the product; they actually know it even better than consumers. So, this is more like fake reviews that are left by experts rather than by people who did not try the product. We're trying to see how they would be different from a review by a layperson who actually tried the product. We'll see where this brings us.
We're also trying to see if we can educate consumers to detect fake reviews. People are aware, it's just that they can't do anything about it. We are unable to cognitively engage ourselves in reading and at the same time analyzing the linguistic features of reviews. It's too much for our poor brain.